Integration & Quadrature#
First, let’s recall a few definitions. An indefinite integral is an integral without bounds, and is defined up to a constant \begin{equation} \int x, dx = \frac{x^2}{2} + C \end{equation} A definite integral has bounds, which are sometimes symbolic \begin{equation} \int_0^y 1, dx = y \end{equation}
Symbolic Integration#
sympy
includes a variety of functionality for integration.
import sympy as sym
x, y = sym.symbols('x y')
You can compute indefinite integrals
sym.integrate(1, x)
sym.integrate(x**2 + x - 1)
sym.integrate(x*y, y)
If you want to compute definite integrals, you can pass in the bounds
sym.integrate(1, (x, 0, 2))
sym.integrate(sym.sin(x), (x, 0, sym.pi))
sym.integrate(x*y, (y, 0, 1))
sym.integrate(x, (x, 0, y))
SymPy also provides integral transforms such as the Fourier transform
k = sym.Symbol('k')
sym.fourier_transform(sym.exp(-x**2), x, k)
You can find more complete information in the sympy documentation
Numerical Integration (Quadrature)#
Numerical integration is used to obtain definite integrals.
First, we should recall the definition of the Riemannian integral: \begin{equation} \int_a^b f(x), dx = \lim_{n\to \infty} \frac{b-a}{n}\sum_{i=1}^n f(x_i) (x_{i+1} - x_i) \end{equation} where the sequence \(\{x_i\}\) is built for each \(n\) so that \(x_{i+1} - x_{i} = (b-a)/n\). It is useful to think of how integrals compute area under a curve. In this case, we are (approximately) computing this area as the sum of rectangles with height \(f(x_i)\) and width \(x_{i+1}-x_i\). More generally, we can think of this as a weighted sum of function values \begin{equation} \sum_{i=1}^n f(x_i) w_i \end{equation} Which looks more like Lesbegue integration, where \(w_i\) is the measure assigned to the point \(x_i\).
In order to numerically integrate functions, we will take a finite sum of weighted function values, where the weights and function evaluation points are designed to give a good approximation to the integral. This is known as quadrature (instead of inegration).
Trapezoidal Rule#
One of the simplest quadrature rules is trapezoid rule, which approximates an area under a curve by trapezoids defined by the four points \((x_i,0), (x_{i+1},0), (x_i, f(x_i)), (x_{i+1}, f(x_{i+1}))\). The area of this trapezoid is \begin{equation} \frac{1}{2}\bigg(f(x_{i+1}) + f(x_i)\bigg)(x_{i+1} - x_i) \end{equation} If we have \(a = x_0 \le x_1 \le ... \le x_{n} = b\) equally spaced, then the quadrature weights for \(x_i\) are \(w_i = (b-a)/n\) if \(i\notin \{0,n\}\), and \(w_i= (b-a)/(2n)\) if \(i \in \{0,n\}\)
You can use trapezoid rule in scipy
using scipy.integrate.trapz
import numpy as np
from scipy.integrate import trapz
x = np.linspace(0,1,100)
y = x**2
trapz(y, x) # actual answer is 1/3
0.33335033840084355
Other quadrature rules that you can use easily are Simpson’s rule (simps
) and Romberg’s rule (romb
)
from scipy.integrate import simps
simps(y,x) # again, answer is 1/3
0.3333333333333333
Gaussian Quadrature#
Gaussian quadrature computes points x_i
and weights w_i
to optimally integrate polynomials up to some fixed order. This is all wrapped up in fixed_quad
or quadrature
functions
from scipy.integrate import fixed_quad, quadrature
f = lambda x: x**10
fixed_quad
integrates up to fixed polynomial order
1/11
0.09090909090909091
fixed_quad(f, 0, 1, n=11) # n is order of polynomial
(0.09090909090909104, None)
The return tuple is the computed result and None
.
quadrature
integrates up to a fixed tolerance
quadrature(f, 0, 1)
(0.09090909090909097, 1.249000902703301e-16)
for ifunction in (fixed_quad, quadrature):
print(ifunction(f, 0, 1)[0])
0.09090765936004021
0.09090909090909097
The return tuple is the computed result and the error, measured as the difference between the last two estimates.
General Quadrature#
The function quad
wraps a varitety of functionality. Basic use is just like above
from scipy.integrate import quad
f = lambda x: x**2
quad(f, 0, 1)
(0.33333333333333337, 3.700743415417189e-15)
Again, the function returns the computed result, and an estimate of the absolute error.
You can also compute integrals on unbounded domains
f = lambda x: np.exp(-x**2)
quad(f, 0, np.inf)
(0.8862269254527579, 7.101318390472462e-09)
Functions with Discontinuities#
Discontinuities can cause problems with any sort of quadrature scheme that assumes functions are continuous. You can specify problematics points with points
import matplotlib.pyplot as plt
f = lambda x : np.floor(x**2)
x = np.linspace(0,2,100)
plt.plot(x, f(x))
plt.show()
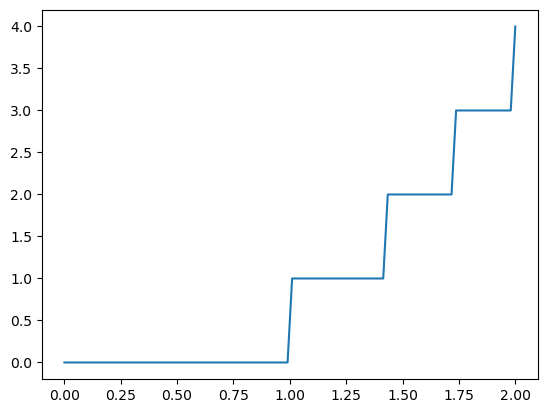
quad(f, 0, 2)
(1.8537356322944702, 9.277312917888025e-09)
quad(f, 0, 2, points=np.sqrt(np.arange(5)))
(1.8537356300580277, 2.0580599780584437e-14)
Functions with Singularities#
Depending on the type of singularity, you can pass in different arguments into the weight
and wvar
parameters of quad
. See the documentation for details.
For example, let’s say we want to compute
\begin{equation} \int_{-1}^2 \frac{1}{x},dx \end{equation}
f = lambda x : 1 / x
x = np.linspace(-1,2,100)
plt.plot(x, f(x))
plt.show()
/tmp/ipykernel_1199/3625872093.py:1: RuntimeWarning: divide by zero encountered in divide
f = lambda x : 1 / x
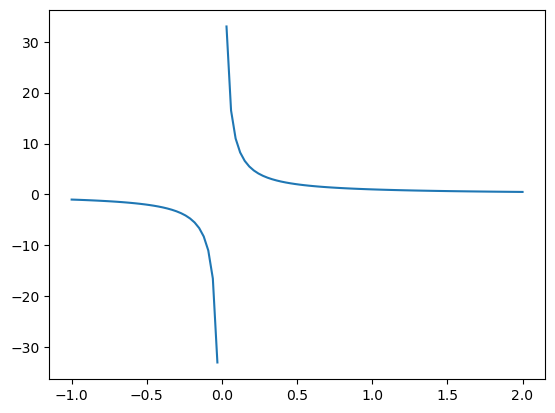
There is an odd symmetry in the interval \([-1,1]\), so we can calculate
\begin{equation} \int_{-1}^2 \frac{1}{x},dx = \int_1^2 \frac{1}{x},dx = \log(2) - \log(1) = \log(2) \end{equation}
The 'cauchy'
weight will calculate the integral of \(\frac{f(x)}{x - {\tt wvar}}\) (i.e. we will weight the fuction \(f\) by the Cauchy distribution \(\frac{1}{x - {\tt wvar}}\)).
So we can use weight='cauchy'
with wvar=0
on the function f(x) = 1
to compute our integral.
quad(lambda x : 1, -1, 2, weight='cauchy', wvar=0) # mulitply function by 1/x and do integration
(0.6931471805599452, 0.0)
np.log(2)
0.6931471805599453
Multivariate Integration#
You can integrate over functions of multiple variables using
nquad
integrates over a hyper cube defined by ranges in the second argument. dblquad
and tplquad
can allow you to integrate over more complicated domains.
from scipy.integrate import nquad
f = lambda x, y, z : np.exp( -x**2 - y**2 - z**2)
nquad(f, ((-3,3), (-3,3), (-3,3)))
(5.56795898358481, 1.4605401208842099e-08)
Monte Carlo Integration#
Monte Carlo integration is a method which computes integrals by taking a sum over random samples.
\begin{equation} \int_{a}^b f(x) = \mathbb{E}_{U(a,b)} [f] \end{equation}
Where \(U(a,b)\) is the uniform distribution over the interval \([a,b]\). We can estimate this expected value by drawing samples from the distribution, and computing
\begin{equation} \mathbb{E}{U(a,b)} [f] \approx \frac{1}{N} \sum{i=1}^N f(x_i), x_i \sim U(a,b) \end{equation}
Or, more generally, we might define an integral over a probability measure \(\mu\)
\begin{equation} \int f(x), d\mu(x) = \mathbb{E}{\mu} [f] = \frac{1}{N} \sum{i=1}^N f(x_i), x_i \sim \mu(x) \end{equation}
The expected error can be derived from the law of large numbers, and is typically \(\frac{1}{\sqrt{N}}\). In high dimensions, this is typically the most effictive way to integrate functions, as quadrature requires a number of samples that is exponential in terms of the dimension.
Example#
The classic example of Monte Carlo integration is computing \(\pi\) using random samples. Recall the area of the unit circle is \(\pi\), and the area of the square \([-1,1]^2\) is \(4\). We sample points uniformly from this square, and compute how many fall in the unit circle - the expected ratio of points inside the circle to the total number of points is \(\pi/4\).
def compute_pi_mc(n=1000):
x = np.random.rand(n,2)*2 - 1 # sampled in [-1,1] x [-1,1]
r = np.linalg.norm(x, axis=1) # computes radius of each point
return 4 * np.sum(r <= 1.) / n
%time compute_pi_mc(10_000_000)
CPU times: user 261 ms, sys: 121 ms, total: 381 ms
Wall time: 381 ms
3.1403216
We can use numba to parallelize this
from numba import njit
@njit
def compute_pi_mc_numba(n=1000):
x = np.random.rand(n,2)*2 - 1
ct = 0
for i in range(n):
ct += x[i,0]**2 + x[i,1]**2 < 1
return 4 * ct / n
%time compute_pi_mc_numba(10_000_000)
CPU times: user 684 ms, sys: 90.6 ms, total: 774 ms
Wall time: 778 ms
3.1412964
from numba import njit, prange
@njit(parallel=True)
def compute_pi_mc_numba_parallel(n=1000):
x = np.random.rand(n,2)*2 - 1
ct = 0
for i in prange(n):
ct += x[i,0]**2 + x[i,1]**2 < 1
return 4 * ct / n
%time compute_pi_mc_numba_parallel(10_000_000)
CPU times: user 1.26 s, sys: 314 ms, total: 1.58 s
Wall time: 835 ms
3.1419732
if we look at the wall time, we see this runs faster than any of the other options