Symbolic Computing#
Symbolic computing allows you to use a computer to do all the algebra you might otherwise do by hand.
SymPy is a popular package for symbolic computing.
conda install sympy
import sympy as sym
import math
import numpy as np
A good place to start is the SymPy tutorial
Let’s compare how sympy
evaluates sqrt
vs. math
print(math.sqrt(8))
print(np.sqrt(8))
2.8284271247461903
2.8284271247461903
sym.sqrt(8)
Note that jupyter notebooks render the output nicely.
the math
package gives a floating point approximation to \(\sqrt{8}\), whereas sympy
simplifies the expression by removing any divisors that are perfect squares.
a = sym.sqrt(8)
print(a)
type(a)
2*sqrt(2)
sympy.core.mul.Mul
Algebraic Expressions#
Symbolic computing is particularly useful for manipulating algebraic expressions.
from sympy import symbols, expand, factor
You can build expressions using symbols:
x, y = symbols('x y')
expr = 2*x + y
expr
Let’s say we forgot how to mulitply monomials
a, b, c, d = symbols('a b c d')
x, y = symbols('x, y')
ex1 = a*x + b
ex2 = c*x + d
ex1 * ex2
we can expand an expression by multiplying everything out and combining like terms:
ex = expand(ex1 * ex2)
ex
We can also factor an expression that has been expanded:
factor(ex)
You can substitute values (or other symbols) for symbols:
ex.subs([(a, 1), (b, 2), (c, 3), (d, y)])
ex_sub = ex.subs([(a, 1), (b, 2), (c, 2), (d, 3)])
ex_sub
You can evaluate expressions numerically:
ex_sub.evalf(subs={x: 0.1})
SymPy uses arbitrary precision arithmetic. The default precision is 10 decimal digits (between single and double precision), but you can increase the precision in evalf
sym.pi.evalf()
sym.pi.evalf(100)
Symbolic Mathematics#
Sympy supports just about everything you might have learned in courses on algebra and calculus (and more)
import sympy as sym
Differentiation#
expr = sym.sin(x)
print(expr)
print(sym.diff(expr)) # symbolic differentiation w.r.t x
sin(x)
cos(x)
expr = x * y
sym.diff(expr, x) # differentiate w.r.t. x
Integration#
expr = sym.sin(x)
print(expr)
print(sym.integrate(expr, x)) # indefinite integral w.r.t x
sin(x)
-cos(x)
the sympy variable oo
(two o
symbols) is used to represent \(\infty\)
from sympy import oo
expr = sym.exp(-(x**2))
sym.integrate(expr, (x, -oo, oo)) # integration with limits
sym.integrate(expr, (x, -1, 1))
Limits#
You can compute limits. For example: \begin{equation} \lim_{x\to 0} \frac{\sin(x)}{x} \end{equation}
sym.limit(sym.sin(x)/x, x, 0) # limit as x \to 0
Roots#
You can compute roots of functions using solve
(i.e. we solve the system f(x) = 0
for x
)
sym.solve(x**2 - 3, x)
[-sqrt(3), sqrt(3)]
Symbolic to Numerical Functions#
You can turn sympy functions into lambda functions that are compatible with numpy using lambdify
:
f = sym.sin(x)
g = sym.lambdify(x, f, 'numpy')
g(np.array(np.linspace(0,1,10)))
array([0. , 0.11088263, 0.22039774, 0.3271947 , 0.42995636,
0.52741539, 0.6183698 , 0.70169788, 0.77637192, 0.84147098])
Plotting Functions#
You can plot a symbolic function using the sympy plot
function
f = sym.sin(x)
sym.plot(f)
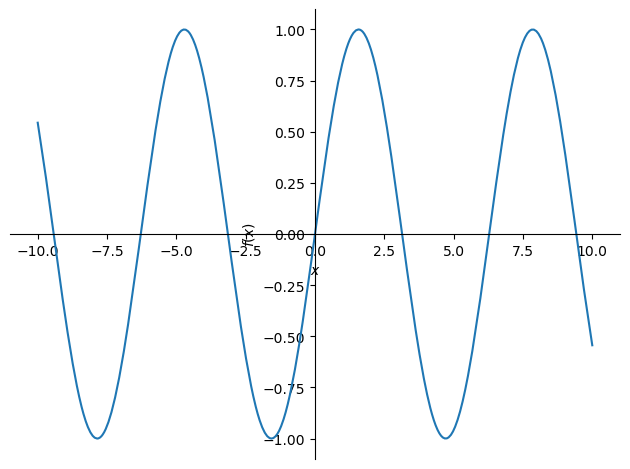
<sympy.plotting.plot.Plot at 0x7f91e02a3f10>
you can change the domain using a tuple with the variable and lower and upper bounds
sym.plot(f, (x, -5,5))
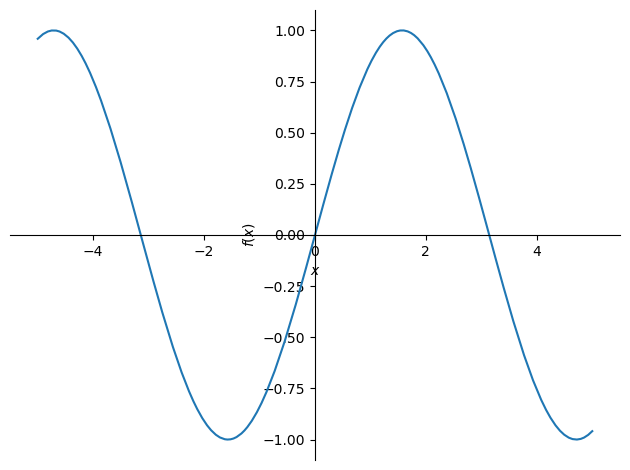
<sympy.plotting.plot.Plot at 0x7f91de74bd50>
There are a variety of keyword arguments you can use for formatting as well. See help(sym.plot)
for more information.
Exercises#
Use sympy to compute solutions to answer the following questions
What is \(\int_{1}^{\infty} x^{-2}\, dx\)?
Find a value of
x
wheresin(x) = cos(x)
sym.integrate((x)**(-2), (x, 1, oo))
from sympy.solvers import solve
from sympy import *
x = Symbol('x')
solve(sin(x)-cos(x),x)
[pi/4]