Distances#
A common task when dealing with data is computing the distance between two points.
We can use scipy.spatial.distance
to compute a variety of distances.
import numpy as np
import scipy.linalg as la
import matplotlib.pyplot as plt
import scipy.spatial.distance as distance
A data set is a collection of observations, each of which may have several features. We’ll consider the situation where the data set is a matrix X
, where each row X[i]
is an observation. We’ll use n
to denote the number of observations and p
to denote the number of features, so X
is a \(n \times p\) matrix.
For example, we might sample from a circle (with some gaussian noise)
def sample_circle(n, r=1, sigma=0.1):
"""
sample n points from a circle of radius r
add Gaussian noise with variance sigma^2
"""
X = np.random.randn(n,2)
X = r * X / np.linalg.norm(X,axis=1).reshape(-1,1)
return X + sigma * np.random.randn(n,2)
X = sample_circle(100)
plt.scatter(X[:,0], X[:,1])
plt.show()
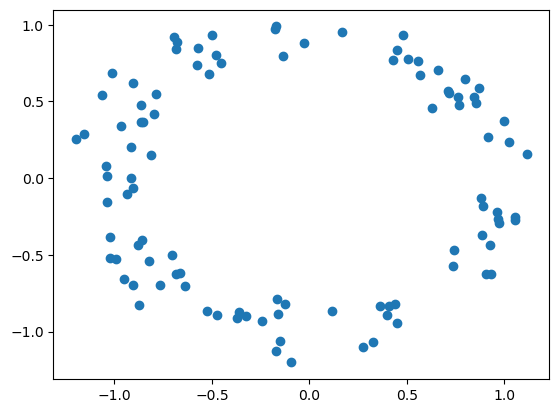
We might also call a data set a “point cloud”, or a collection of points in some space.
Similarity, Dissimilarity, and Metric#
A similarity between two points \(x, y\in X\) is a function \(s: X \times X \to \mathbb{R}\), where \(s\) is larger if \(x,y\) are more similar.
Cosine similarity is an an example of similarity for points in a real vector space. We can define it as \begin{equation} c(x,y) = \frac{x^T y}{|x|_2 |y|_2} \end{equation}
Note \(c(x,x) = 1\), and if \(x,y\) are orthogonal, then \(c(x,y) = 0\).
A dissimilarity between two points \(x,y \in X\) is a function \(d: X \times X \to \mathbb{R}_+\), where \(d\) is smaller if \(x,y\) are more similar. Sometimes, disimilarity functions will be called distances.
Cosine distance is an example of a dissimilarity for points in a real vector space. It is defined as \begin{equation} d(x,y) = 1 - c(x,y) \end{equation} Note \(d(x,x) = 0\), and \(d(x,y) = 1\) if \(x,y\) are orthogonal.
A metric is a disimilarity \(d\) that satisfies the metric axioms
\(d(x,y) = 0\) iff \(x=y\) (identity)
\(d(x,y) = d(y,x)\) (symmetry)
\(d(x,y) \le d(x,z) + d(z,y)\) (triangle inequality)
You are probably most familiar with the Euclidean metric \begin{equation} d(x,y) = |x - y|_2 \end{equation}
Exercise#
Write functions for the cosine similarity, cosine distance, and euclidean distance between two numpy arrays treated as vectors.
## Your code here
Solution:#
Computing Distances#
SciPy provides a variety of functionality for computing distances in scipy.spatial.distance
.
x = [1.0, 0.0]
y = [0.0, 1.0]
distance.euclidean(x, y) # sqrt(2)
1.4142135623730951
distance.cosine(x, y)
1.0
the \(L_1\) / Manhattan / City block distance \begin{equation} d(x,y) = |x - y|_1 = \sum_i |x_i - y_i| \end{equation}
distance.cityblock(x, y)
2.0
The \(L_\infty\) / Chebyshev distance \begin{equation} d(x,y) = |x - y|_\infty = \max_i |x_i - y_i| \end{equation}
distance.chebyshev(x, y)
1.0
More generally, the Minkowski distance \begin{equation} d(x,y) = |x - y|_p = \big( \sum_i (x_i - y_i)^p \big)^{1/p} \end{equation}
distance.minkowski(x, y, 3)
1.2599210498948732
np.cbrt(2) # cube root of 2
1.2599210498948734
The Mahalanobis distance. Note that this is defined in terms of an inverse covariance matrix. In fact, you can use it to compute distances based on the \(A\)-norm where \(A\) is any symmetric positive definite (SPD) matrix.
\begin{equation} d(x,y) = | x- y |_A = \sqrt{(x - y)^T A (x - y)} \end{equation}
A = np.random.randn(3,2)
A = A.T @ A
distance.mahalanobis(x, y, A)
3.791565791818502
You can also weight many distances with a vector \(w\). The entry \(w_i\) will weight the comparison between the \(i\)th entries of \(x\) and \(y\). E.g. weighted euclidean distance \begin{equation} d(x, y; w) = \sqrt{\sum_i w_i(x_i - y_i)^2} \end{equation}
w = [1, 2]
for d in (distance.euclidean, distance.cityblock, lambda x, y, w : distance.minkowski(x, y, 3, w)):
print(d(x, y, w))
1.7320508075688774
3.0
1.4422495703074083
Exercise#
Compute a weighted euclidean distance using the Mahalanobis distance.
## Your code here
Pairwise Distances#
In general, we often want to compute distances between many points all at once. This typically boils down to computing a matrix \(D\), where \(D_{i,j} = d(x_i, x_j)\).
Here’s a simple function that would do just that
def pairwise(X, dist=distance.euclidean):
"""
compute pairwise distances in n x p numpy array X
"""
n, p = X.shape
D = np.empty((n,n), dtype=np.float64)
for i in range(n):
for j in range(n):
D[i,j] = dist(X[i], X[j])
return D
X = sample_circle(5)
pairwise(X)
array([[0. , 0.30319957, 0.34105369, 1.83236742, 1.88826178],
[0.30319957, 0. , 0.11891106, 1.87895696, 1.99089783],
[0.34105369, 0.11891106, 0. , 1.76894693, 1.89124471],
[1.83236742, 1.87895696, 1.76894693, 0. , 0.37151272],
[1.88826178, 1.99089783, 1.89124471, 0.37151272, 0. ]])
pairwise(X, dist=lambda x, y : distance.minkowski(x, y, 3, [1.0, 2.0]))
array([[0. , 0.32255029, 0.41071762, 1.78252772, 1.822932 ],
[0.32255029, 0. , 0.12235965, 1.81110967, 1.94994064],
[0.41071762, 0.12235965, 0. , 1.70670453, 1.85986066],
[1.78252772, 1.81110967, 1.70670453, 0. , 0.39851746],
[1.822932 , 1.94994064, 1.85986066, 0.39851746, 0. ]])
There are a couple of inneficiencies above:
D is symmetric (we do redundant computation)
For some metrics, it can be more efficient to compute many distances at once
As an example of point 2, pairwise euclidean distances are \begin{equation} d(x_i, x_j) = \sqrt{(x_i - x_j)^T (x_i -x_j)}\ = \sqrt{x_i^T x_i - 2 x_i^T x_j + x_j^T x_j} \end{equation}
Let \(x^2\) denote the vector of length \(n\) where \(x_i^2 = x_i^T x_i\), and let \(1\) denote the vector of ones (of length \(n\)). Then \begin{equation} D = \sqrt{1 x^{2T} + x^2 1^T - 2 X X^T} \end{equation}
Thus, we can take advantage of BLAS level 3 operations to compute the distance matrix
def pairwise_euclidean(X):
"""
Compute pairwise euclidean distances in X
"""
XXT = X @ X.T
x2 = np.diag(XXT)
one = np.ones(X.shape[0])
D = np.outer(x2, one) + np.outer(one, x2) - 2*XXT
return np.sqrt(D)
la.norm(pairwise_euclidean(X) - pairwise(X))
1.1571112726691442e-15
We can also use BLAS syr2 for the rank-2 update:
print(la.blas.dsyr2.__doc__)
a = dsyr2(alpha,x,y,[lower,incx,offx,incy,offy,n,a,overwrite_a])
Wrapper for ``dsyr2``.
Parameters
----------
alpha : input float
x : input rank-1 array('d') with bounds (*)
y : input rank-1 array('d') with bounds (*)
Other Parameters
----------------
lower : input int, optional
Default: 0
incx : input int, optional
Default: 1
offx : input int, optional
Default: 0
incy : input int, optional
Default: 1
offy : input int, optional
Default: 0
n : input int, optional
Default: ((len(x)-1-offx)/abs(incx)+1 <=(len(y)-1-offy)/abs(incy)+1 ?(len(x)-1-offx)/abs(incx)+1 :(len(y)-1-offy)/abs(incy)+1)
a : input rank-2 array('d') with bounds (n,n)
overwrite_a : input int, optional
Default: 0
Returns
-------
a : rank-2 array('d') with bounds (n,n)
def pairwise_euclidean_blas(X):
"""
Compute pairwise euclidean distances in X
use syrk2 for rank-2 update
"""
XXT = X @ X.T
x2 = np.diag(XXT)
one = np.ones(X.shape[0])
D = la.blas.dsyr2(1.0, x2, one, a=-2*XXT) # this only updates upper triangular part
D = np.triu(D) # extract upper triangular part
return np.sqrt(D + D.T)
la.norm(pairwise_euclidean_blas(X) - pairwise(X))
1.0569008315563939e-15
import time
# generate data set
n = 100
d = 2
X = np.random.randn(n, d)
for d in (pairwise, pairwise_euclidean, pairwise_euclidean_blas):
t0 = time.time()
D = d(X)
t1 = time.time()
print("{} : {} sec.".format(d.__name__, t1 - t0))
pairwise : 0.041265249252319336 sec.
pairwise_euclidean : 0.004239559173583984 sec.
pairwise_euclidean_blas : 0.00018477439880371094 sec.
Exercise#
Write a version of pairwise_euclidean
which uses Numba. How does this compare to the BLAS implementation?
## Your code here
pdist
#
You don’t need to implement these faster methods yourself. scipy.spatial.distance.pdist
has built-in optimizations for a variety of pairwise distance computations. You can use scipy.spatial.distance.cdist
if you are computing pairwise distances between two data sets \(X, Y\).
from scipy.spatial.distance import pdist, cdist
D = pdist(X)
The output of pdist
is not a matrix, but a condensed form which stores the lower-triangular entries in a vector.
D.shape
(4950,)
to get a square matrix, you can use squareform
. You can also use squareform
to go back to the condensed form.
from scipy.spatial.distance import squareform
D = squareform(D)
D.shape
(100, 100)
squareform(D).shape
(4950,)
pdist
takes in a keyword argument metric
, which determines the distance computed
pdist(X, metric='cityblock')
array([2.37331677, 2.06840507, 1.71775182, ..., 5.00535973, 1.82927861,
3.38074781])
Let’s compare to our implementations
import time
# generate data set
n = 100
d = 2
X = np.random.randn(n, d)
for d in (pairwise, pairwise_euclidean, pairwise_euclidean_blas, pdist):
t0 = time.time()
D = d(X)
t1 = time.time()
print("{} : {} sec.".format(d.__name__, t1 - t0))
pairwise : 0.04546785354614258 sec.
pairwise_euclidean : 0.0004761219024658203 sec.
pairwise_euclidean_blas : 0.00013256072998046875 sec.
pdist : 4.220008850097656e-05 sec.
If we form the squareform:
import time
# generate data set
n = 100
d = 2
X = np.random.randn(n, d)
def sq_pdist(X, **kwargs):
return squareform(pdist(X, **kwargs))
for d in (pairwise, pairwise_euclidean, pairwise_euclidean_blas, sq_pdist):
t0 = time.time()
D = d(X)
t1 = time.time()
print("{} : {} sec.".format(d.__name__, t1 - t0))
pairwise : 0.04576921463012695 sec.
pairwise_euclidean : 0.0004417896270751953 sec.
pairwise_euclidean_blas : 0.00012636184692382812 sec.
sq_pdist : 6.4849853515625e-05 sec.
D = cdist(X[:30],X[30:])
D.shape
(30, 70)
Exercise#
Use pdist
to compute ’braycurtis’, ‘canberra’, ‘chebyshev’, ‘cityblock’, ‘correlation’, ‘cosine’, and ‘euclidean’, distances on a random X
. Are there noticeable differences in the time it takes to compute each distance?
## Your code here