Nearest Neighbors#
Given a point cloud, or data set \(X\), and a distance \(d\), a common computation is to find the nearest neighbors of a target point \(x\), meaning points \(x_i \in X\) which are closest to \(x\) as measured by the distance \(d\).
Nearest neighbor queries typically come in two flavors:
Find the
k
nearest neighbors to a pointx
in a data setX
Find all points within distance
r
from a pointx
in a data setX
There is an easy solution to both these problems, which is to do a brute-force computation
Brute Force Solution#
import numpy as np
import matplotlib.pyplot as plt
import scipy as sp
import scipy.spatial
import scipy.spatial.distance as distance
n = 1000
d = 2
X = np.random.rand(n,d)
plt.scatter(X[:,0], X[:,1])
plt.show()
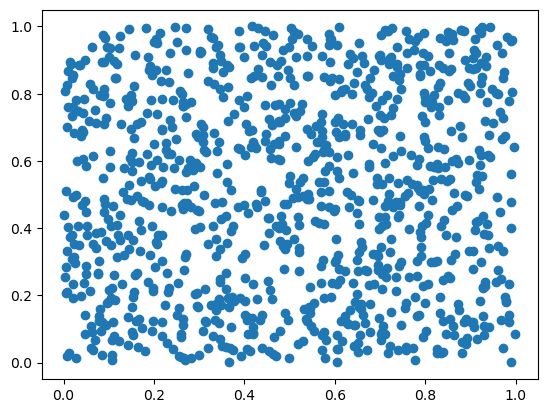
def knn(x, X, k, **kwargs):
"""
find indices of k-nearest neighbors of x in X
"""
d = distance.cdist(x.reshape(1,-1), X, **kwargs).flatten()
return np.argpartition(d, k)[:k]
x = np.array([[0.5,0.5]])
inds = knn(x, X, 50)
plt.scatter(X[:,0], X[:,1], c='b')
plt.scatter(X[inds,0], X[inds,1], c='r')
plt.show()
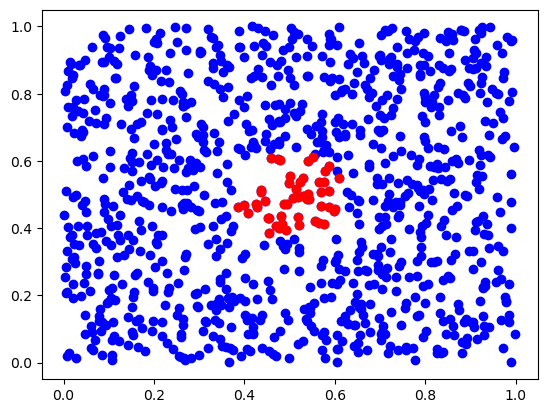
def rnn(x, X, r, **kwargs):
"""
find r-nearest neighbors of x in X
"""
d = distance.cdist(x.reshape(1,-1), X, **kwargs).flatten()
return np.where(d<r)[0]
inds = rnn(x, X, 0.2)
plt.scatter(X[:,0], X[:,1], c='b')
plt.scatter(X[inds,0], X[inds,1], c='r', label="neighbors")
plt.legend()
plt.show()
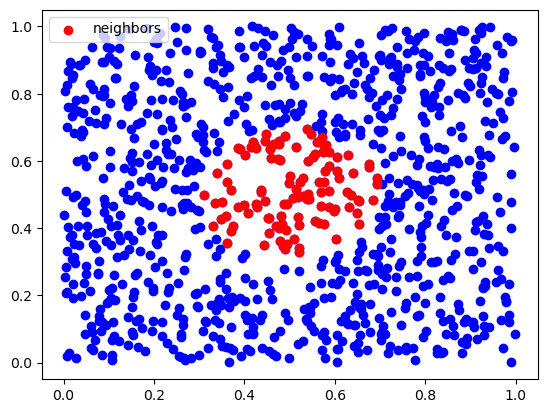
Exercise#
What is the time complexity of both the above functions?
show your work
KD-trees#
One of the issues with a brute force solution is that performing a nearest-neighbor query takes \(O(n)\) time, where \(n\) is the number of points in the data set. This can become a big computational bottleneck for applications where many nearest neighbor queries are necessary (e.g. building a nearest neighbor graph), or speed is important (e.g. database retrieval)
A kd-tree, or k-dimensional tree is a data structure that can speed up nearest neighbor queries considerably. They work by recursively partitioning \(d\)-dimensional data using hyperplanes.
scipy.spatial
provides both KDTree
(native Python) and cKDTree
(C++). Note that these are for computing Euclidean nearest neighbors
from scipy.spatial import KDTree, cKDTree
tree = KDTree(X)
ds, inds = tree.query(x, 50) # finds 50-th nearest neighbors
plt.scatter(X[:,0], X[:,1], c='b')
plt.scatter(X[inds,0], X[inds,1], c='r')
plt.show()
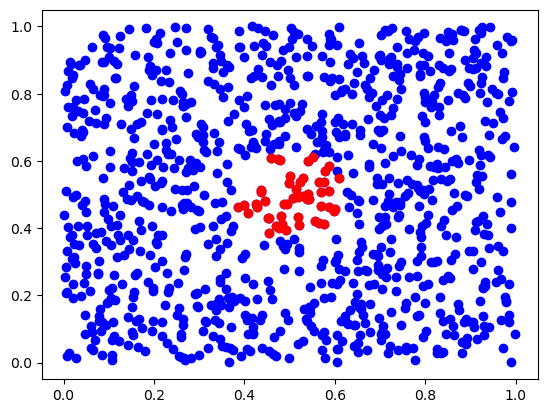
inds = tree.query_ball_point(x, 0.2) # finds neighbors in ball of radius 0.1
inds = inds[0]
plt.scatter(X[:,0], X[:,1], c='b')
plt.scatter(X[inds,0], X[inds,1], c='r')
plt.show()
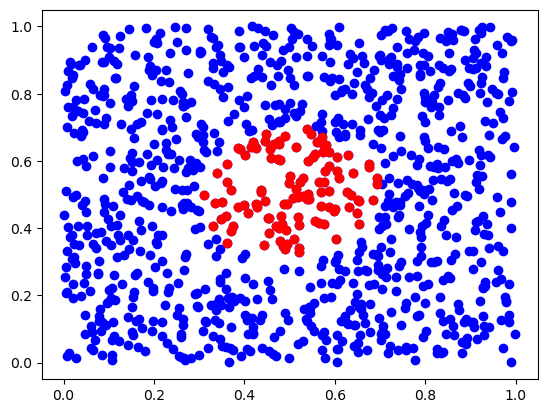
cKDTrees
have the same methods
ctree = scipy.spatial.cKDTree(X)
ds, inds = ctree.query(x, 50) # finds 50-th nearest neighbors
plt.scatter(X[:,0], X[:,1], c='b')
plt.scatter(X[inds,0], X[inds,1], c='r')
plt.show()
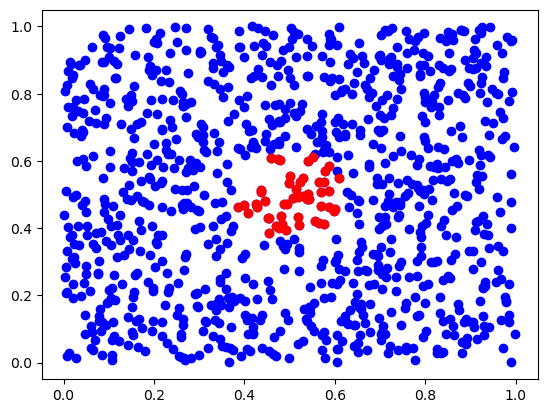
inds = tree.query_ball_point(x, 0.1) # finds neighbors in ball of radius 0.1
inds = inds[0]
plt.scatter(X[:,0], X[:,1], c='b')
plt.scatter(X[inds,0], X[inds,1], c='r')
plt.show()
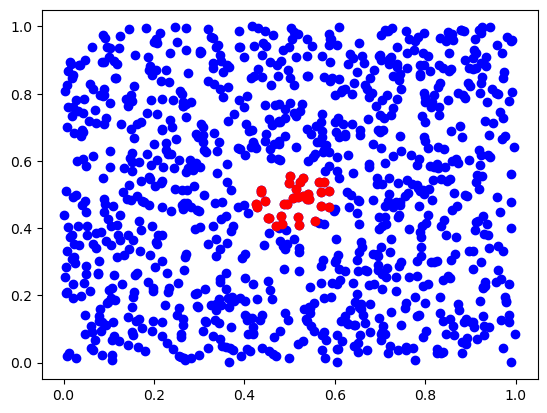
Performance Comparision#
import time
k=50
n = 100000
d = 2
Y = np.random.rand(n,d)
t0 = time.time()
inds = knn(x, Y, 50)
t1 = time.time()
print("brute force: {} sec".format(t1 - t0))
t0 = time.time()
tree = KDTree(Y)
ds, inds = tree.query(x, 50) # finds 50-th nearest neighbors
t1 = time.time()
print("KDTree: {} sec".format(t1 - t0))
t0 = time.time()
ds, inds = tree.query(x, 50) # finds 50-th nearest neighbors
t1 = time.time()
print(" extra query: {} sec".format(t1 - t0))
t0 = time.time()
tree = cKDTree(Y)
ds, inds = tree.query(x, 50) # finds 50-th nearest neighbors
t1 = time.time()
print("cKDTree: {} sec".format(t1 - t0))
t0 = time.time()
ds, inds = tree.query(x, 50) # finds 50-th nearest neighbors
t1 = time.time()
print(" extra query: {} sec".format(t1 - t0))
brute force: 0.0015192031860351562 sec
KDTree: 0.030165433883666992 sec
extra query: 0.00010848045349121094 sec
cKDTree: 0.02585148811340332 sec
extra query: 9.942054748535156e-05 sec
Ball trees#
If you want to do nearest neighbor queries using a metric other than Euclidean, you can use a ball tree. Scikit learn has an implementation in sklearn.neighbors.BallTree
.
KDTrees take advantage of some special structure of Euclidean space. Ball Trees just rely on the triangle inequality, and can be used with any metric.
from sklearn.neighbors import BallTree
---------------------------------------------------------------------------
ModuleNotFoundError Traceback (most recent call last)
Cell In[15], line 1
----> 1 from sklearn.neighbors import BallTree
ModuleNotFoundError: No module named 'sklearn'
The list of built-in metrics you can use with BallTree
are listed under sklearn.neighbors.DistanceMetric
tree = BallTree(X, metric="minkowski", p=np.inf)
for a k-nearest neighbors query, you can use the query
method:
ds, inds = tree.query(x, 500)
plt.scatter(X[:,0], X[:,1], c='b')
plt.scatter(X[inds,0], X[inds,1], c='r')
plt.show()
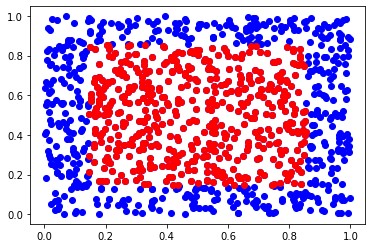
for r-nearest neighbors, you use query_radius
instead of query_ball_point
.
inds = tree.query_radius(x, 0.2)
inds = inds[0]
plt.scatter(X[:,0], X[:,1], c='b')
plt.scatter(X[inds,0], X[inds,1], c='r')
plt.show()
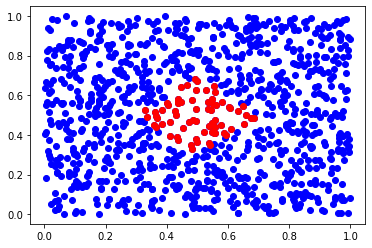
tree = BallTree(X, metric='chebyshev')
inds = tree.query_radius(x, 0.15)
inds = inds[0]
plt.scatter(X[:,0], X[:,1], c='b')
plt.scatter(X[inds,0], X[inds,1], c='r')
plt.show()
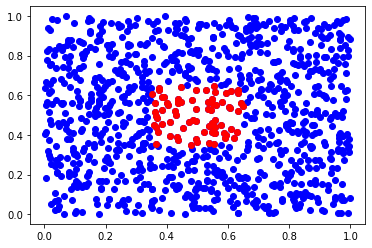
Exercises#
Compare the performance of
KDTree
,cKDTree
, andBallTree
for doing nearest neighbors queries in the Euclidean metricScikit learn also has a
KDTree
implementation:sklearn.neighbors.KDTree
- how does this compare to the KDTree implementations in scipy?
## Your code here